Interactive Labeling
The Machine Learning approaches, which are currently perceived as successful, are in many cases based on supervised learning.
To train such models, large amounts of labeled data are required. The provision of the labels is a major challenge which often prevents the application of ML in productive environments where labels are not necessarily available. While proposing different approaches, the following underlying research question is addressed:
How can we efficiently label training data with an interactive interplay of ML model and expert knowledge ?
Sequence of unsupervised learning and autoencoder-based clearning of clusters
Cluster-Clean-Label: An interactive Machine Learning approach for labeling high-dimensional data.
Beil, D. and Theissler, A. (2020). 13th International Symposium on Visual Information Communication and Interaction (VINCI 2020). Association for Computing Machinery (ACM), ISBN: 978-1-4503-8750-7.
Download , Link to paper
Combining Active Learning and user guidance
VisGIL: Machine Learning based Visual Guidance for Interactive Labeling,
Benedikt Grimmeisen, Mohammad Chegini, Andreas Theissler (2022),
Springer, The Visual Computer
Open access: Link to paper
The Machine Learning Model as a Guide: Pointing Users to Interesting Instances for Labeling through Visual Cues.
Grimmeisen, B. and Theissler, A. (2020). 13th International Symposium on Visual Information Communication and Interaction (VINCI 2020). Association for Computing Machinery (ACM), ISBN: 978-1-4503-8750-7.
Download , Link to paper
Visual Interactive Labelling for Anomaly Detection
VIAL-AD: Visual Interactive Labelling for Anomaly Detection – An approach and open research questions.
Andreas Theissler, Anna-Lena Kraft, Max Rudeck, Fabian Erlenbusch (2020). Proceedings of the Workshop on Interactive Adaptive Learning co-located with European Conference on Machine Learning and Principles and Practice of Knowledge Discovery in Databases (ECML PKDD 2020) pages 84-89, CEUR, Vol-2660, ISSN 1613-0073.
Link to paper
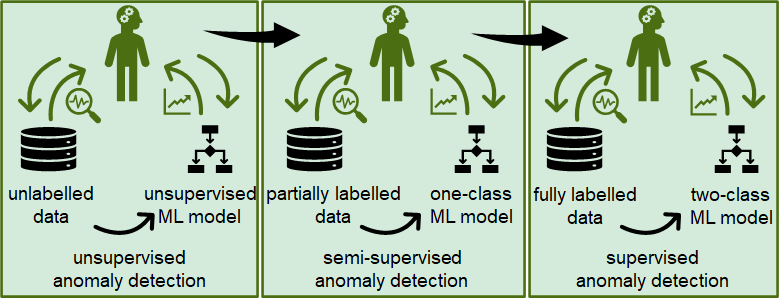