Research Topic: Anomaly Detection
We propose ROCKAD, a kernel-based approach for semi-supervised whole time series anomaly detection, i.e. the assignment of a single anomaly score to an entire time series.
Our key idea is to use the time series classifier ROCKET as an unsupervised feature extractor and to train anomaly detectors to deduce an anomaly score. To the best of our knowledge, this is the first approach to transfer the ideas of ROCKET to the task of anomaly detection.
The approach addresses whole time series anomaly detection:
Definition: Semi-supervised whole time series anomaly detection is the assignment of a single anomaly score alpha to an entire time series xT by an anomaly detector MAD, i.e. „whole series“. MAD is trained on a training set solely containing instances from the normal class, i.e. „semi-supervised“.
Source Code on GitHub:
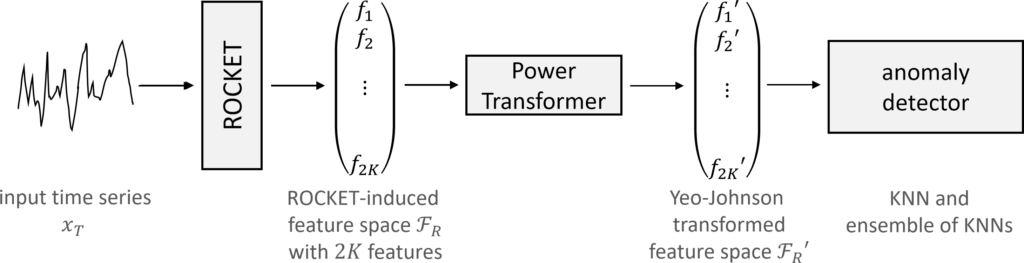
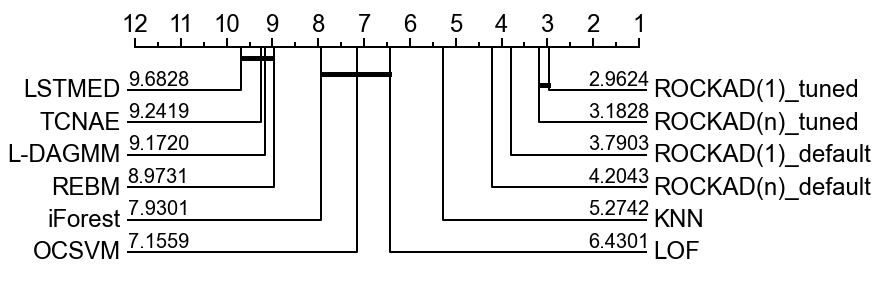
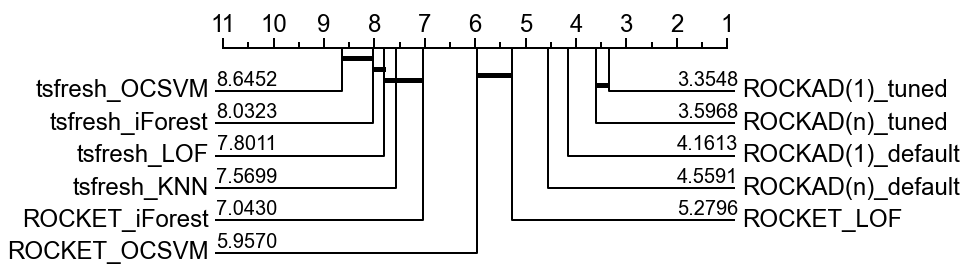
Conference paper:
Theissler, Andreas, Manuel Wengert, and Felix Gerschner. „ROCKAD: Transferring ROCKET to Whole Time Series Anomaly Detection.“ Advances in Intelligent Data Analysis XXI: 21st International Symposium on Intelligent Data Analysis, IDA 2023. Cham: Springer Nature Switzerland, 2023.
Link to paper
Detailed result table:
Table of results on UCR time series data sets (adapted to the problem of anomaly detection, see paper for details):